Germany
July 2021
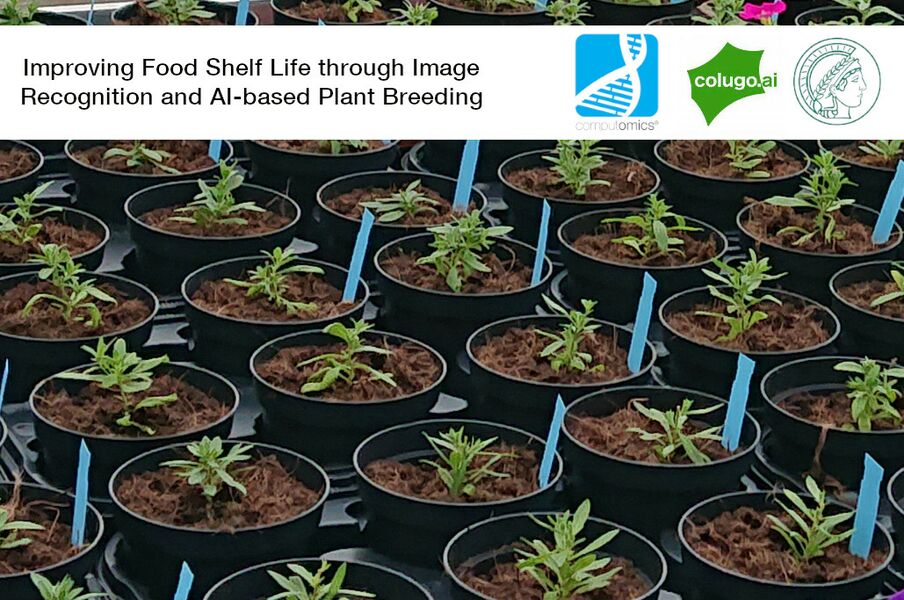
Together with academic partner Max Planck Institute for Developmental Biology and commercial partner Colugo, a team at Computomics evaluated how a plant shelf life test can be predicted by using AI methods. Plants were provided by Selecta for evaluation. The project was funded by the Ministry of Economy, Labor and Housing of Baden-Württemberg and was successfully concluded in May 2021.
The funded project is part of the Innovation Competition Artificial Intelligence for SME of the state of Baden-Württemberg.
Challenge
1.3 billion tons of food are wasted globally each year, roughly a third of all food produced. Approximately 60 percent of the food waste is simply food that is past its shelf life and is not fit for consumption anymore. Fruits and vegetables have a relatively short shelf life. Breeding new varieties of fruits and vegetables with increased shelf life can help alleviate the issue of food waste.
Project Goal
Reducing the cost of plant breeding to make it more attractive to small and mid-sized enterprises (SME) and enable breeding of plants with increased shelf life.
Applying artificial intelligence (AI) methods and genome research to
- develop automated procedures for plant scoring based on image recognition to supplement or replace the manual scoring, which is labor intensive (and prone to error), and
- identify genetic markers related to increased shelf life.
Approach and Analysis Plan
- Collect Image Data
- Plant phenotype scoring
- Reduced-representation sequencing
We collected data for 1,500 plants:
200 Capsicum genotypes (“varieties”) of bell peppers, chili plants, with 3 replicates each
– Collection of 2 leaf samples per genotype for sequencing
– Pictures and manual scores for all replicates at 4 time points, up to 11 features per time point
– 400 leaf samples, 2,400 photos, rating of up to 26,400 features

300 Calibrachoa genotypes (“varieties”), 3 replicates each
– Collection of 2 leaf samples per genotype for sequencing
– Pictures and manual scorings for all replicates at 4 time points, up to 11 features per time point
– 600 leaf samples, 3,600 photos, rating of up to 39,600 features
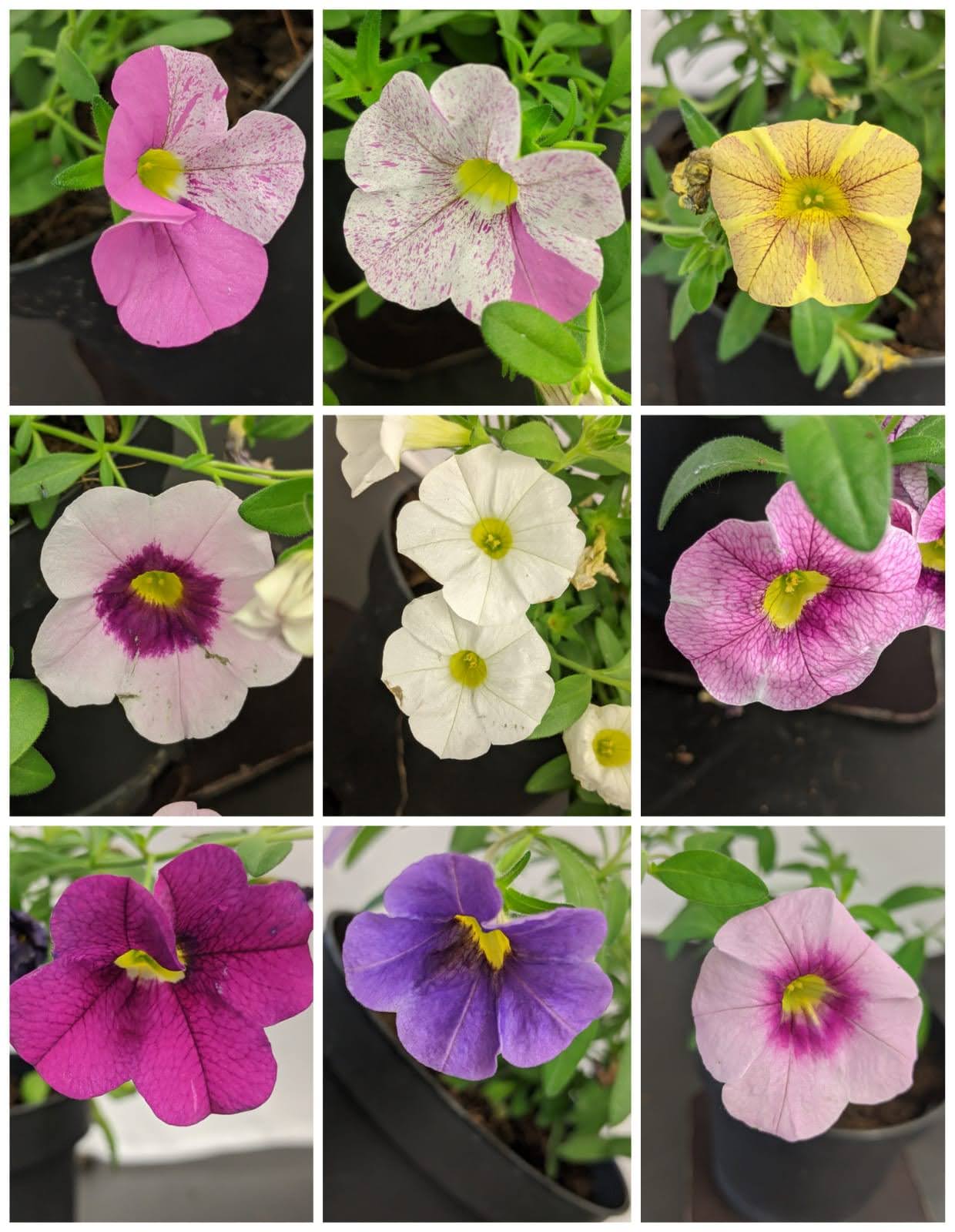
Results
Data Collection Phase
In the data collection phase, more than 6,000 high-resolution images have been taken (approx. 200 GBytes) as basis for machine learning. Plant scoring generated approx. 25,000 data points for machine learning and genotype/phenotype associations. 1,000 leaf samples were taken for DNA sequencing and genotyping.
Plant Scoring
Score prediction via deep learning methods works well and is giving a fast prediction result. Improvements with more and better training data is expected.
Genotyping
Scoring prediction using machine learning is an established service at Computomics. Genetic marker candidates associated with shelf life have been identified.
Next Steps
Machine learning delivered sufficient accuracy for predicting scoring results. Thus, one of the main goals of the project has been achieved and we have come much closer to the goal of automating the assessment. The next step is to integrate the AI-based assessment as another analysis module into CropScore, Computomics' automated phenotyping system. This would not only further increase efficiency, but also enable the use of much larger data sets to be used to improve the accuracy of the AI models.
For more details of the project or how to implement CropScore, please reach out to us.
Consortium Members:
Computomics GmbH
Computomics brings its experience in the field of camera systems with image recognition of plant characteristics and their assignment to environmental conditions and genetic breeding markers to the project.
Colugo GmbH
Colugo develops and implements solutions in the field of image data processing and artificial intelligence. The focus is on modern AI processes such as deep learning and other machine learning methods, such as kernel regression and gradient boosting.
Max Planck Institute (MPI) for Developmental Biology
The MPI for Developmental Biology is a leading global research location for plant research, genetics and evolutionary biology. The cooperating department is a leader in molecular biology, sequencing and bioinformatics and is experienced in assigning breeding marker-environment interaction.
Non-funded:
Selecta Klemm GmbH und Co&KG
For additional information visit Wirtschaft digital BW (German).
In case of question reach out to us.