Germany
January 2021
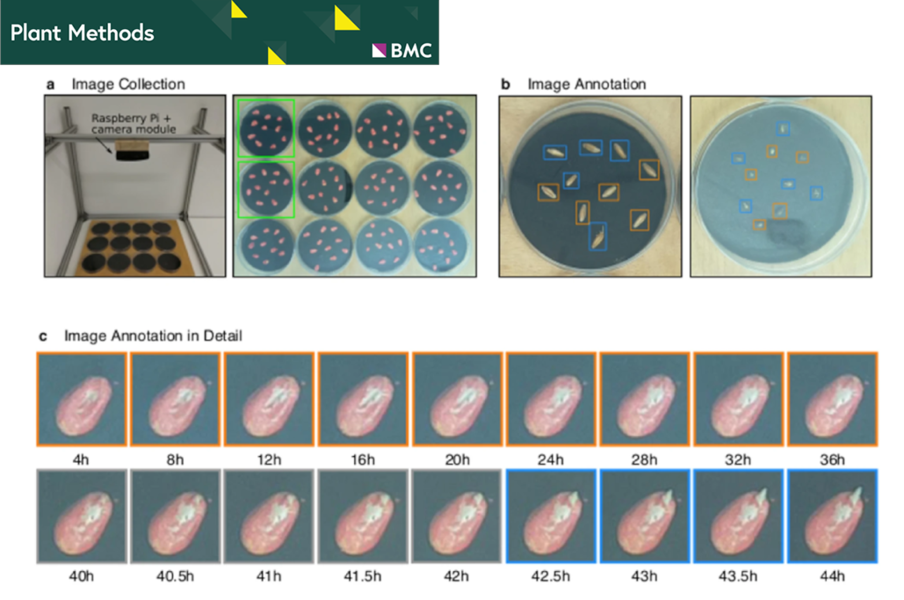
In the publication authored by Nikita Genze, Richa Bharti, Michael Grieb, Sebastian J. Schultheiss and Dominik G. Grimm, the researchers evaluated the germination process of more than 2,400 seeds of three different crops, Zea mays (maize), Secale cereale (rye) and Pennisetum glaucum (pearl millet), with more than 23,000 images in total.
Computomics' CropScore System was used to capture all images to document and evaluate the germination process of the three crops. Seed Germination was captured over a time span of two days with a resolution of one image per half hour. Check out the publication's abstract below.
Abstract
Background
Assessment of seed germination is an essential task for seed researchers to measure the quality and performance of seeds. Usually, seed assessments are done manually, which is a cumbersome, time consuming and error-prone process. Classical image analyses methods are not well suited for large-scale germination experiments, because they often rely on manual adjustments of color-based thresholds. We here propose a machine learning approach using modern artificial neural networks with region proposals for accurate seed germination detection and high-throughput seed germination experiments.
Results
We generated labeled imaging data of the germination process of more than 2400 seeds for three different crops, Zea mays (maize), Secale cereale (rye) and Pennisetum glaucum (pearl millet), with a total of more than 23,000 images. Different state-of-the-art convolutional neural network (CNN) architectures with region proposals have been trained using transfer learning to automatically identify seeds within petri dishes and to predict whether the seeds germinated or not. Our proposed models achieved a high mean average precision (mAP) on a hold-out test data set of approximately 97.9%, 94.2% and 94.3% for Zea mays, Secale cereale and Pennisetum glaucum respectively. Further, various single-value germination indices, such as Mean Germination Time and Germination Uncertainty, can be computed more accurately with the predictions of our proposed model compared to manual countings.
Conclusion
Our proposed machine learning-based method can help to speed up the assessment of seed germination experiments for different seed cultivars. It has lower error rates and a higher performance compared to conventional and manual methods, leading to more accurate germination indices and quality assessments of seeds.
Computomics co-founder Dr. Sebastian J. Schultheiss is proud to be a Co-Author of this publication.
Click here to access the full publication.